Learn the characteristics of data quality and metrics you should track.
In 1967, the largest and most modern oil tanker in the world ran aground on a reef off of the U.K.
The Torrey Canyon caused the largest maritime environmental disaster the world had seen to date and one of the key reasons was bad measurements. The captain and crew did not know precisely where they were in relationship to the reef. With high quality data, collected and presented in the right way, you can effectively plan, make decisions, and know what is happening in your operating environment (and avoid catastrophic reefs).
The quality of your data is intricately connected to your organization’s ability to reach goals and solve challenges. Data won’t be useful—it won’t be able to serve its purpose—unless it’s high quality. Keep reading and we’ll explain more.
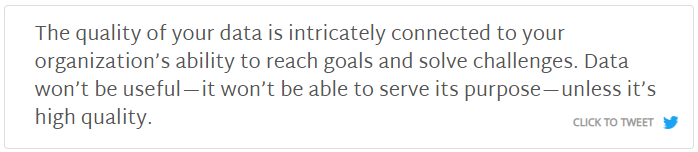
Why Data Quality Is Important
The quality of your data is important because it directly affects your strategic decision making. Poor quality data results in poor decisions that can drain time and money. In fact, IBM estimated that poor quality data cost the company $3.1 trillion in the U.S. alone in 2016.
Conversely, high quality data leads to smart decisions that help organizations succeed. Companies that commit to improving their data quality have proven to increase revenue by 15% to 20%. This “commitment to improvement” centers on determining how to accurately measure data quality, as well as taking action to improve both your data and how your organization uses it. This can help you raise the level of everything from customer service and profits to team morale.
So, how can you differentiate between good and bad? How do you know the quality of your data? There are seven standard characteristics, or dimensions, of quality. If you understand these characteristics and develop metrics to track them, you’ll have your answers.
7 Characteristics Of Data Quality & Metrics To Track
The elements of data quality and example metrics below can act as yardsticks for determining the value of your information.
1. Consistency
Data has no contradictions in your databases. This means that if two values are examined from separate data sets, they will match or align. For example, the budget amount for a specific department needs to be consistent across the organization so as not to exceed its total budget. In many cases, you may be looking to established data rules to verify consistency.
Examples of consistency metrics:
- Range
- Variance
- Standard deviation
2. Accuracy
Data is error-free and exact. Accuracy is when a measured value matches the actual (true) value and it contains no mistakes, such as outdated information, redundancies, and typos. Your goal is to continually increase the accuracy of your data, even as your datasets grow in size.
Examples of accuracy metrics:
- Error ratio
- Deviation
3. Completeness
Data records are “full” and contain enough information to draw conclusions. Tracking this data quality metric involves finding any fields that contain missing or incomplete values. All data entries must be complete in order to compose a high quality data set.
Examples of completeness metrics:
- Percentage of data records that contain all needed information
4. Auditability
Data is accessible and changes are traceable. Can you drill down into your data and see a history of updates? Determining quality with regard to this metric means tracking the percentage of fields where you cannot determine what and when edits were made, and by whom.
Examples of auditability metrics:
- Percentage of gaps in the data set
- Percentage of altered data
- Percentage of disassociated data
- Percentage of untraceable data
5. Validity
Data points exist in the same and correct format everywhere they appear. This can also be called data integrity. Having a high rate of validity means that all data aligns with your established formatting rules—such as rounding percentages to the nearest whole number or formatting dates as mm/dd/yyyy. You can track validity by comparing the number of format errors for a data item to the number of times that data appears in total across your databases.
Examples of validity metrics:
- Percentage of data records where all values are in the required format
6. Uniqueness
Data will be recorded no more than once. This doesn’t mean you can’t use the same data point in multiple ways—such as a quarterly revenue number appearing in both a sales and leadership report—but more that there aren’t erroneous duplicates. For example, the same initiative can’t be listed twice under a goal. Tracking this metric helps organizations identify and avoid double data entry.
Examples of uniqueness metrics:
- Number or percentage of repeated values
7. Timeliness
Data is available and accurate. It’s important to collect data in a timely manner in order to effectively track changes. If you’re expecting a project to immediately impact a measure, track the measure on a monthly basis, versus annually. You also shouldn’t have to revise data several months later. Although, this can admittedly be tricky in certain situations, such as with medical outcomes from patients trying new treatments or medicine.
Examples of timeliness metrics:
- Time variance
Keep in mind that improving the quality of your data is a continual process rather than a one-time job. All your data quality metrics should improve over time, but it won’t happen instantly. Your goal is to keep quality trending upward, without faltering.
FAQ:
What are data quality checks?
Data quality checks are processes or procedures used to assess and ensure the accuracy, completeness, consistency, and reliability of data. These checks involve validating data against predefined criteria or rules to detect errors, anomalies, or discrepancies that may impact data quality.
What are data quality rules?
Data quality rules are predefined guidelines or criteria used to assess the quality of data. These rules define acceptable and unacceptable conditions for data based on specific attributes, such as accuracy, completeness, consistency, timeliness, and validity. By applying data quality rules, organizations can enforce standards and improve the reliability and usefulness of their data.
What are data quality tools?
Data quality tools are software applications or platforms designed to monitor, assess, and improve the quality of data within an organization. These tools automate data profiling, cleansing, enrichment, and monitoring processes to ensure data meets defined quality standards. Examples include data profiling tools, data cleansing software, master data management (MDM) systems, and data quality dashboards.
What are data quality dimensions?
Data quality dimensions refer to specific aspects or characteristics used to evaluate the quality of data. Common data quality dimensions include:
- Accuracy: How close data values are to their true or intended values.
- Completeness: The extent to which data is whole, including all required parts or records.
- Consistency: The absence of contradictions or discrepancies between different data sources or elements.
- Timeliness: The availability of data when needed and its relevance within a specific timeframe.
- Validity: The conformity of data to defined business rules, formats, or standards.
Assessing data quality across these dimensions helps organizations identify areas for improvement and ensure data is fit for its intended use.
What are data quality issues?
Data quality issues encompass any problems or challenges that affect the reliability, accuracy, or usability of data. Common data quality issues include incomplete or missing data, inaccurate data entries, duplicate records, inconsistent formats or standards, outdated information, and lack of data governance. Addressing these issues is crucial for maintaining trustworthy data that supports informed decision-making and operational efficiency.